How AI can help you boost quality and productivity
Generally speaking, artificial intelligence (AI) refers to technologies that support and strengthen human capabilities in terms of seeing, hearing, analysing, decision-making and acting. Today, the most well-known and promising ones are neural networks that can be used to automate image, sound and vibration analyses, among others. These abstracted models of artificial, networked neurons can, for instance, be used for defect identification or predictive maintenance. As a section of IT, data science has at its disposal methods for extracting knowledge from structured and unstructured data and finding the right AI tool for the respective application. In this process, data and process analysis are at the beginning of every decision on if and how AI can be used for the respective application.
Great potential and various applications
Artificial intelligence can be used in almost every industry, provided sufficient data is available. AI-based applications have been part of the consumer product world for some time already, for instance in smart phones with facial recognition or camera-based assistance systems in cars. High-performance language processing models such as GPT-3 or Chat GPT that use artificial intelligence and machine learning create texts that are almost indistinguishable from texts written by humans. Linked to the capability of generating images from textual descriptions, such technologies harbour great potential.
Identifying complex relationships in data
Many sectors of industry are looking into the possibilities of artificial intelligence. Production processes are particularly well suited for the use of AI as they usually produce large quantities of data that is highly usable and mark a good base for AI applications. Additionally, processes such as quality assurance are an important and multi-faceted topic in production. This is where AI can identify complex relationships in data flows – relationships that humans are incapable of recognising – and derive rules from them. These algorithms support the optimisation and automation of quality assurance processes and can significantly contribute to their efficiency and cost-reduction.
Trends such as automated image recognition and analysis offer a basis for other methods that are well suited for use in industry, such as anomaly recognition. In industrial production, these can be used for automatically checking components for their quality during the production process. Using pre-determined training images, the AI algorithm learns what a component should look like. During the production process, a camera supplies images of a component which are then analysed, allowing for defective components to be identified. The large benefit of the anomaly detection approach is that deviations from a desired target component state are detected, eliminating the need for all possible defects to be known from the start. The calculation models of neural networks reach their limits when decisions need to be transparent and describable. Transparency plays a crucial role in applications such as autonomous driving. In the event of an accident, for instance, it must be entirely transparent whether the driver or the car's ECU was responsible or why the system made a certain decision. Explainable AI deals with this very dilemma, aiming at explaining solution approaches and thus increasing the transparency and acceptance of AI.
Crucial expertise, process and data analysis
Whether the use of AI makes sense and what solution matches the respective application needs to be decided upon following a comprehensive analysis of the process in question and the available data. In practice, several iterations are often required as additional data sources are often discovered or new information or newly added aspects must be assessed and the AI solution must be adapted. In the course of this iterative process, the calculation model is constantly improved to achieve an optimum result. There are a lot of standard AI solutions, but most only match a single use case. More complex and specific applications require individual customisation and the expertise of an IT expert, to develop a successful solution following the analysis of data and processes. Seamless integration of the AI solution, for example in the production process and machine control, is a key success factor. Likewise, data storage in a data warehouse requires broad IT expertise.
Added value, purpose and limitations
The use of AI tools requires the availability of data in digital form. The more historic data is available the better the calculation models and analysis that can be derived from it. Legal regulations such as data privacy guidelines and the performance of the hardware used are among the limitations of AI applications. Cameras taking pictures of hundreds of products per minute on a production line for quality assurance require lots of computing power and storage space. Furthermore, there are many situations which require 100 percent accuracy or zero tolerance, for instance in the production of medicinal products. Nonetheless, AI solutions already generate high value: Whenever processes can be largely digitised or automated with the help of AI, it proves highly useful and saves resources and human labour. The use of AI not only proves useful in production, but also in many other areas. Automated classification of documents achieves an average success rate of 80 – 99 percent.
Higher quality and productivity
The biggest advantages of artificial intelligence lie in the technology’s constant and reliable performance when it comes to recognising complex relationships in data – relationships that would remain hidden to humans – and in faster decision-making. This consistency is particularly important in quality assurance, for example. Contrary to a human worker, AI tools never tire and often complete their tasks much faster than any human ever could. Intelligent systems can thus be used to boost quality and productivity. Data analysis also creates a significant added value by making information usable and drawing conclusions from it. Condensed in an AI model, this knowledge creates new potential – for instance by uncovering previously unknown relationships between various process steps and making them usable. In any case, artificial intelligence offers a mighty tool. The future will see more and more systems that “assist” people, making tasks easier or supplying additional information.
About the author:
In his role as a Machine Learning Consultant, Matthias Puhr supports and advises customers in implementing projects involving artificial intelligence, machine learning and data science. Possessing many years of experience and a broad technical background, he develops optimum solutions for complex problems.
Get in touch!
We are looking forward to your message
* Please fill out all fields
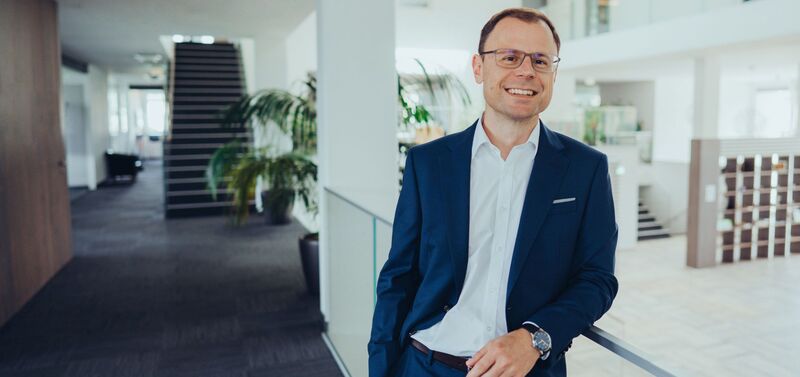