Business Analytics
Successfully utilising the hype
According to a study* involving 267 companies in the DACH region, 72 per cent are looking into Advanced Analytics prototypes, while 28 per cent are already making such solutions operational within their processes. Advanced Analytics, Big Data and Data Science, without a doubt, offer companies great potential. But what are the essential prerequisites and experiences as well as challenges for companies connected to their implementation?
Focus on data and processes
Business Analytics always needs a structured data warehouse as well as high data quality and availability as a basis in order to analyse and use this digital gold. Without a qualified database, even self-service approaches to data analysis are doomed to fail. However, a suitable, agile corporate culture that allows experimentation and failure is also part of the prerequisites. At the beginning of a BA project there should be simple use cases, similar to those used by start-ups, which allow an idea to be tested without a lot of budget and time - according to the motto "Sometimes you win, sometimes you learn". Interaction between process and data managers is important in this context. That’s because interdisciplinary teams and working hypotheses help understand processes and data flows and to develop an algorithm – such as a forecast model – from this.
High expectations hinder progress
In practise, excessively high expectations and lack of experience hinder progress in the abstraction of business processes and the development of business analytics applications. Artificial intelligence, for instance, is a lot more complex than a black box that is fed with data and spits out a solution. First, data needs to be available at high quality and quantity to be able to use algorithms properly. Also fundamental for successful BA projects and detailed process analysis is well-maintained master or machine data. Data protection regulations also often have a hindering effect. In industry in particular, it is hardly ever personal data, but rather data from machines that should be made transparent and used. If you want to offer predictive maintenance services, for example, you have to continuously record the condition of the machine via sensors in order to be able to plan maintenance pro-actively.
Step by step towards success
Generally speaking, one should proceed in small steps according to the “crawl, walk, run” method. A well-structured data warehouse is a must to start structuring, preparing, analysing and enriching data. Next, one needs to create a scalable platform, preferably cloud-based, that allows to incorporate additional data sources such as environmental and device information. Platforms such as SAP and Microsoft that are open and flexible, also have proven successful. After that, one can develop and incorporate Advanced Analytics prototypes into the process. In this step, it is important to start simple, experiment, learn quickly and respond with flexibility. Keep in mind that algorithms are not absolute truths, but statistic methods that include deviations. Experienced service providers accompanying the entire project and offering competent support with specific problems, can help with implementation.
Great savings potential
Business Analytics offers a large range of user potential – especially in industry and production, but also for the service and administration sectors. Artificial intelligence and machine learning applications help you with your work and with saving time and money, when seamlessly embedded into your company’s business processes. Insurance companies, for instance, have started developing algorithms early on to allow fully automated claims processing. The potential is vast – you only have to make the first step.
Stefan Pirer, who wrote this article, is the manager of the DCCS Business Analytics Team
* BARC Usage Study of Advanced Analytics 2019
Get in touch!
We are looking forward to your message
* Please fill out all fields
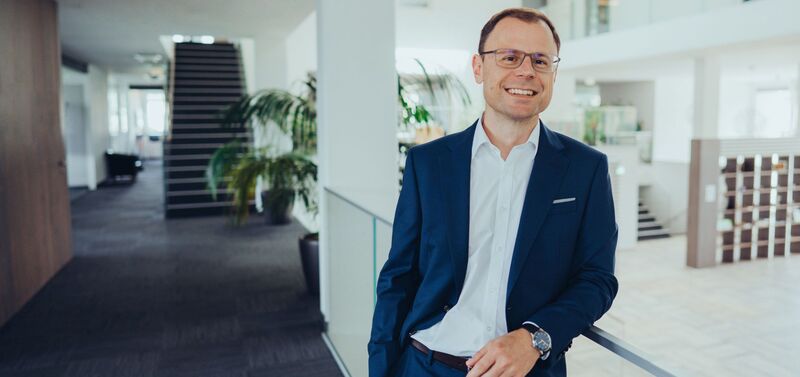